AWS Research on Specializing Large Language Models: Leveraging Self-Talk and Automated Evaluation Metrics for Enhanced Training
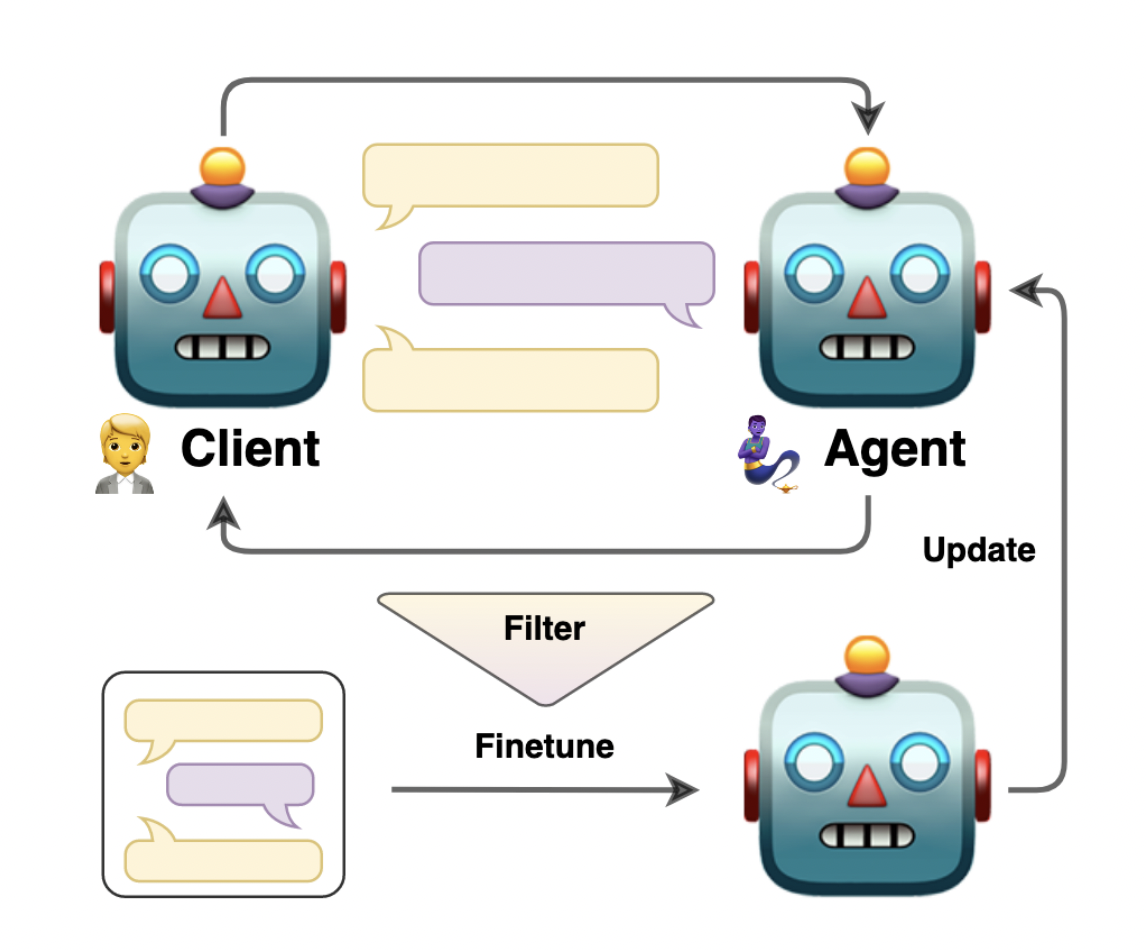
In user-centric applications like personal assistance and customer support, language models are increasingly being deployed as dialogue agents in the rapidly advancing domain of artificial intelligence. These agents are tasked with understanding and responding to various user queries and tasks, a capability that hinges on their ability to adapt to new scenarios quickly. However, customizing these general language models for specific functions presents significant challenges, primarily due to the need for extensive, specialized training data.
Traditionally, the fine-tuning of these models, known as instructing tuning, has relied on human-generated datasets. While effective, this approach faces hurdles like the limited availability of relevant data and the complexities of molding agents to adhere to intricate dialogue workflows. These constraints have been a stumbling block in creating more responsive and task-oriented dialogue agents.
Addressing these challenges, a team of researchers from the IT University of Copenhagen, Pioneer Centre for Artificial Intelligence, and AWS AI Labs have introduced an innovative solution: the self-talk methodology. This approach involves leveraging two versions of a language model that engage in a self-generated conversation, each taking on different roles within the dialogue. Such a method not only aids in generating a rich and varied training dataset but also streamlines fine-tuning the agents to follow specific dialogue structures more effectively.
The core of the self-talk methodology lies in its structured prompting technique. Here, dialogue flows are converted into directed graphs, guiding the conversation between the AI models. This structured interaction results in various scenarios, effectively simulating real-world discussions. The dialogues generated from this process are then meticulously evaluated and refined, yielding a high-quality dataset. This dataset is instrumental in training the agents, allowing them to master specific tasks and workflows more precisely.
The efficacy of the self-talk approach is evident in its performance outcomes. The technique has shown significant promise in enhancing the capabilities of dialogue agents, particularly in their relevance to specific tasks. By focusing on the quality of the conversations generated and employing rigorous evaluation methods, the researchers have isolated and utilized the most effective dialogues for training purposes. This has resulted in the development of more refined and task-oriented dialogue agents.
Moreover, the self-talk method stands out for its cost-effectiveness and innovation in training data generation. This approach circumvents the reliance on extensive human-generated datasets, offering a more efficient and scalable solution. The self-talk methodology thus represents a significant leap forward in the field of dialogue agents, opening new avenues for developing AI systems that can handle specialized tasks and workflows with increased effectiveness and relevance.
In conclusion, the self-talk methodology marks a notable advancement in AI and dialogue agents. It showcases an inventive and resourceful approach to overcoming the challenges of specialized training data generation. This method enhances the performance of dialogue agents and broadens the scope of their applications, making them more adept at handling task-specific interactions. As AI systems become more sophisticated and responsive, innovations are crucial in pushing their capabilities to new heights.
Check out the Paper. All credit for this research goes to the researchers of this project. Also, don’t forget to follow us on Twitter. Join our 36k+ ML SubReddit, 41k+ Facebook Community, Discord Channel, and LinkedIn Group.
If you like our work, you will love our newsletter..
Don’t Forget to join our Telegram Channel
Muhammad Athar Ganaie, a consulting intern at MarktechPost, is a proponet of Efficient Deep Learning, with a focus on Sparse Training. Pursuing an M.Sc. in Electrical Engineering, specializing in Software Engineering, he blends advanced technical knowledge with practical applications. His current endeavor is his thesis on “Improving Efficiency in Deep Reinforcement Learning,” showcasing his commitment to enhancing AI’s capabilities. Athar’s work stands at the intersection “Sparse Training in DNN’s” and “Deep Reinforcemnt Learning”.