Creating Multi-View Optical Illusions with Machine Learning: Exploring Zero-Shot Methods for Dynamic Image Transformation
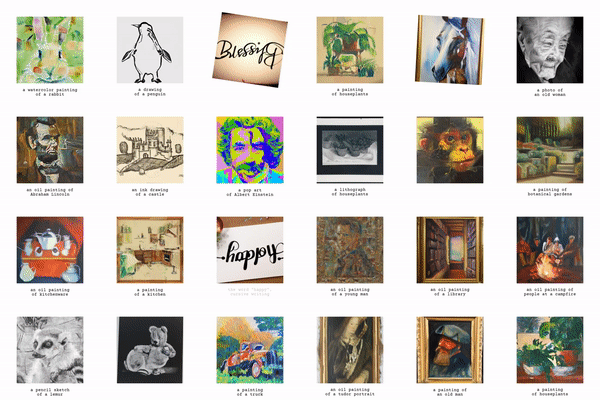
Anagrams are images that change their appearance when you look at them from different angles or flip them around. Creating such illusions usually involves understanding and then tricking our visual perception. However, a new approach has emerged, offering a simple and effective way to generate these captivating multi-view optical illusions.
Many approaches exist for creating optical illusions, but most rely on specific assumptions about how humans perceive images. These assumptions often lead to complex models that may only sometimes capture the essence of our visual experience. Researchers from the University of Michigan have proposed a new solution. Instead of building a model based on how humans see things, it uses a text-to-image diffusion model. This model doesn’t assume anything about human perception; it learns from data alone.
The method introduces a novel way to generate classic illusions, such as images that transform when flipped or rotated. Additionally, it ventures into a new territory of illusions termed “visual anagrams,” where images change appearance when you rearrange their pixels. This encompasses flips, rotations, and more intricate permutations, like creating jigsaw puzzles with multiple solutions, known as “polymorphic jigsaws.” The method even extends to three and four views, broadening the scope of these intriguing visual transformations.
The key to making this method work is carefully selecting views. The transformations applied to the images must preserve the statistical properties of the noise. This is because the model is trained under the assumption of random, independent, and identically distributed Gaussian noise.
The method utilizes a diffusion model to denoise an image from various views, creating multiple noise estimates. These estimates are then combined to form a single noise estimate, facilitating a step in the reverse diffusion process. The paper presents empirical evidence supporting the effectiveness of these views, showcasing both the quality and flexibility of the generated illusions.
In conclusion, this simple yet powerful method opens up new possibilities for creating captivating multi-view optical illusions. By sidestepping assumptions about human perception and leveraging the capabilities of diffusion models, it provides a fresh and accessible approach to the fascinating world of visual transformations. Whether flips, rotations, or polymorphic jigsaws, this method offers a versatile tool for crafting illusions that captivate and challenge our visual understanding.
Check out the Paper and Project. All credit for this research goes to the researchers of this project. Also, don’t forget to join our 33k+ ML SubReddit, 41k+ Facebook Community, Discord Channel, and Email Newsletter, where we share the latest AI research news, cool AI projects, and more.
If you like our work, you will love our newsletter..
Niharika is a Technical consulting intern at Marktechpost. She is a third year undergraduate, currently pursuing her B.Tech from Indian Institute of Technology(IIT), Kharagpur. She is a highly enthusiastic individual with a keen interest in Machine learning, Data science and AI and an avid reader of the latest developments in these fields.