Hudson Labs AI Accelerates And Elevates Equity Research
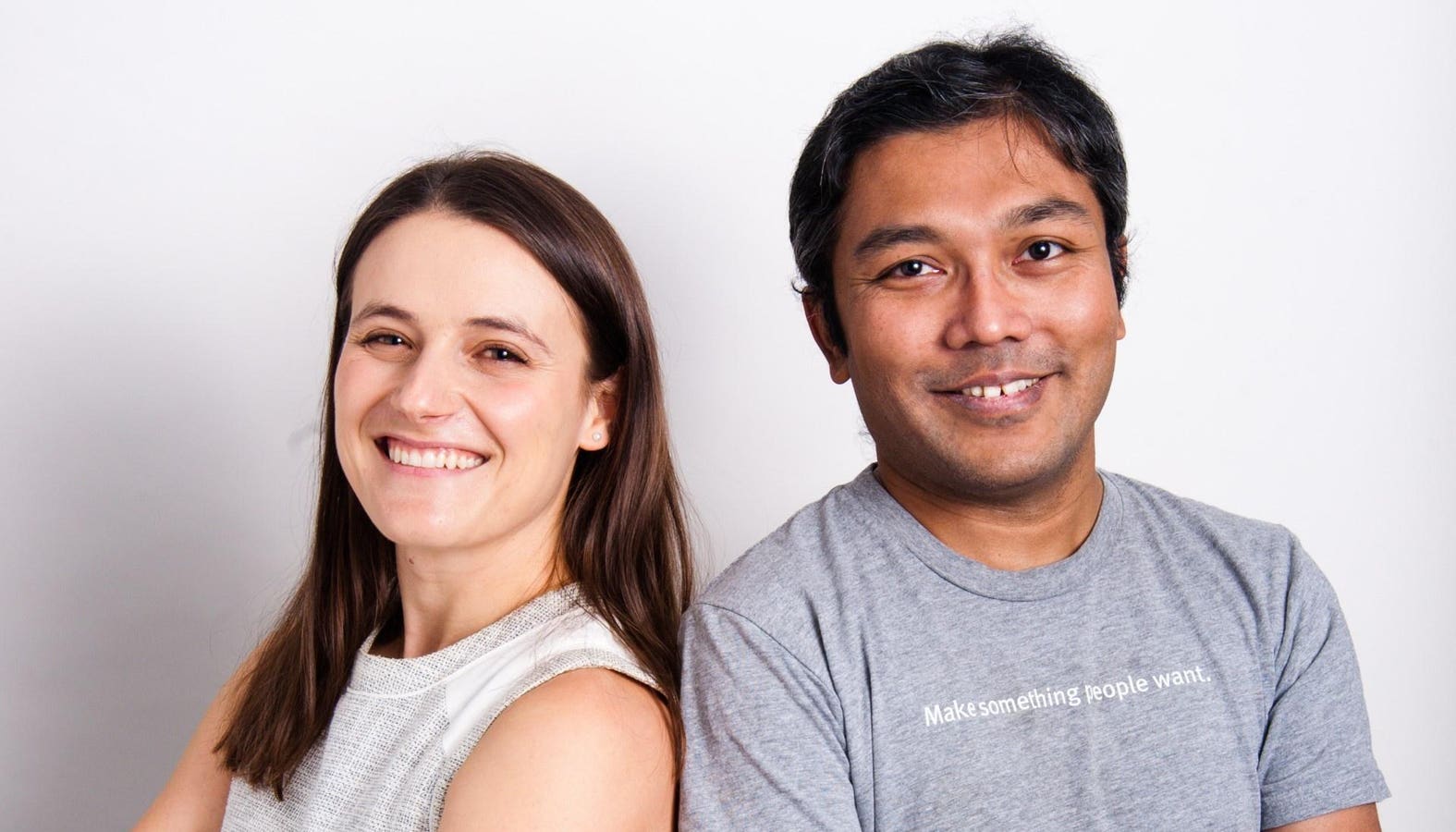
Hudson Labs co-founders CEO Kris Bennatti and CTO Suhas Pai
Hudson Labs
The promise and peril of AI have dominated conversations this year.
As the initial, natural marvel of “chatbots” that instantaneously yield checklists, correspondence and analyses fades, users soon realize that generative AI outputs must be timely, reliable and relevant. Beyond data quality assurance lurks a hushed digital transformation question — will the new tools help or replace jobs?
Equity research provides an ideal setting to examine those challenges. Last year, the SEC received nearly 800,000 regulatory filings. That translates into millions of pages, billions of words and countless figures for analysts’ perusal and dissection.
To address that growing market need, Hudson Labs (formerly known as Bedrock AI), founded in 2019, developed innovative software powered by finance-specific large-language models (LLMs) to automate equity research workflows and extract actionable insights. The firm now serves a client list with over $600 billion in assets under management, including large financial institutions and funds.
Hudson Labs’ platform enables capital markets investment professionals to tap the power of industry-tailored AI. Their success also spotlights three key AI deployment criteria — specialization, trustworthiness and compelling job-acceleration appeal.
Trust And Verify
ChatGPT and other prompt-based generative AI tools have catapulted language modeling into everyday use. Their quick popularity stems from the remarkable ease of simplistic business tasks such as expedited report writing, background research, meeting summaries and transcript keyword extraction.
Across sectors, employers find themselves in a gen AI quandry. The 2024 McLean HR Trends Report found while 79% of surveyed leaders who are deploying gen AI seek increased productivity and efficiency, only 27% of the workforce sees a clear plan for AI’s deployment, use and boundaries.
Further, from a technical perspective, implementation is tricky. Since gen AI is neither a database nor search engine. Popular “generalist” AI models have been “trained” on web data and struggle mightily when sorting through industry-specific, high technical data. Common limitations include “hallucinations” (plausible-sounding false information), reasoning errors and poor output repeatability.
Suhas Pai, Hudson Labs CTO and co-founder, emphasized the importance of contextualizing AI for finance tasks. “LLMs aren’t meant to be a one-size-fits-all solution. Financial text is vastly different from text typically found on the Internet, characterized by financial jargon and legalese, interspersed with numbers, and possessing a distinct linguistic style. Our models have been trained on billions of words of financial text, thus exposing them to financial concepts, textual style and structure, and helping them distinguish between boilerplate and material information.”
Pai explained what distinguishes Hudson Labs’ approach. “Trust and reliability are crucial for an AI product in the financial domain to succeed. Current LLMs suffer from too many issues, including their poor reasoning abilities, propensity to stray away from being factual, and lack of controllability. Instead of using a single LLM end-to-end, we break down a task — like company background memo generation — into dozens of sub tasks. Each subtask is tackled on its own merits, including by using specialized LLMs. This way, we are able to design and deliver highly reliable products that overcome the common limitations of LLMs that persist.”
That solution is essential to difference-making efficiency, relevance and credibility.
Hudson Labs AI Accelerates And Elevates Equity Research
Hudson Labs
Test For Echo
No research analyst can afford to author a factually-wrong report.
Therefore, Hudson Labs put its technology up against a popular gen AI tool and a finance-specific bot in a series of queries about randomly-selected public companies, such as Domino’s Pizza. The experiment relied on well-known, but lesser-followed, market registrants, as the goliaths such as Apple appear more widely in web data.
First, the test asked each platform if seasonality, a common disclosure, affects the selected companies’ business revenues. The “open-forum” bot made up “facts” about seasonality for the sample companies. For instance, for Domino’s, the algorithm reported, “The school year can affect Domino’s sales. Families with children may order more frequently during the school year when they have less time for cooking.” Domino’s, in fact, characterizes its business as “not seasonal” in SEC filings.
Even the more specialized, finance-oriented generative bot also floundered. When asked to list Domino’s reportable business segments, it responded “delivery, carry-out and sit-down.” The correct answer from Domino’s disclosures is “US stores, international stores and supply chain.” Hudson Labs’ AI tools yielded perfect results on all the test queries — a stark contrast to the alternatives’ mixed or failed responses.
That edge is key to improving common equity research tasks.
Hudson Labs CEO and co-founder Kris Bennatti, highlighted, “When analysts worry about their jobs, I remind them that they have to consume vast amounts of information to develop a differentiated view from the rest of the market.”
“If AI makes the process of consuming that information 50% or even 15% easier, their job remains the same with less friction and frustration. For instance, one of Hudson Labs’ contributions to financial AI research is a proprietary noise suppression technique that can be applied to corporate disclosures, call transcripts, etc. In an AI-driven future, you won’t have to read ten pages of nonsense just to find the single point that matters,” she added.
Such perspective exemplifies how curated AI can accelerate and elevate work, calm job replacement fears and underpin meaningful and lasting digital transformation.
Look Ahead
Bennatti sees great prospects in financial services workflow automation. “Moving forward, our finance-specific AI research and technologies enables Hudson Labs to deliver three key timely products — earnings transcript summaries, auditable automated investment memos and AI-generated news feeds for underserved markets.” Such resources can differentiate research and propel top performers.
After all, it’s time to insight, not data, that matters. Who’s ready — or not?