One of the world’s largest AI training datasets is about to get bigger and ‘substantially better’
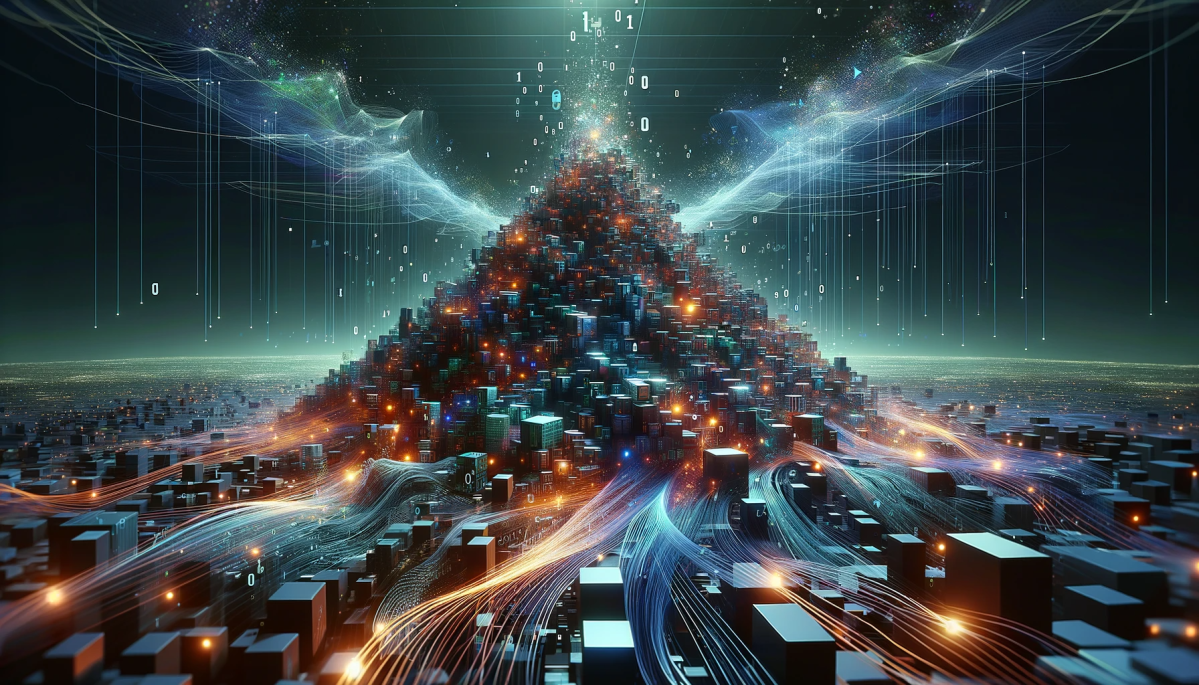
Massive AI training datasets, or corpora, have been called “the backbone of large language models.” But EleutherAI, the organization that created one of the world’s largest of these datasets, an 825 GB open-sourced diverse text corpora called the Pile, became a target in 2023 amid a growing uproar focused on the legal and ethical impact of the datasets that trained the most popular LLMs, from OpenAI’s GPT-4 to Meta’s Llama.
EleutherAI, a grassroots nonprofit research group that began as a loose-knit Discord collective in 2020 that sought to understand how OpenAI’s new GPT-3 worked, was named in one of the many generative AI-focused lawsuits last year. Former Arkansas Governor Mike Huckabee and other authors filed a lawsuit in October that alleged their books were taken without consent and included in Books3, a controversial dataset that contains more than 180,000 works and was included as part of the Pile project (Books3, which was originally uploaded in 2020 by Shawn Presser, was removed from the Internet in August 2023 after a legal notice from a Danish anti-piracy group.)
But far from stopping their dataset work, EleutherAI is now building an updated version of the Pile dataset, in collaboration with multiple organizations including the University of Toronto and the Allen Institute for AI, as well as independent researchers. In a joint interview with VentureBeat, Stella Biderman, a lead scientist and mathematician at Booz Allen Hamilton who is also executive director at EleutherAI, and Aviya Skowron, EleutherAI’s head of policy and ethics, said the updated Pile dataset is a few months away from being finalized.
The new Pile is expected to be bigger and ‘substantially better’
Biderman said that the new LLM training dataset will be even bigger and is expected to be “substantially better” than the old dataset.
“There’s going to be a lot of new data,” said Biderman. Some, she said, will be data that has not been seen anywhere before and “that we’re working on kind of excavating, which is going to be really exciting.”
The Pile v2 includes more recent data than the original dataset, which was released in December 2020 and was used to create language models including the Pythia suite and Stability AI’s Stable LM suite. It will also include better preprocessing: “When we made the Pile we had never trained a LLM before,” Biderman explained. “Now we’ve trained close to a dozen, and know a lot more about how to clean data in ways that make it amenable to LLMs.”
The updated dataset will also include better quality and more diverse data. “We’re going to have many more books than the original Pile had, for example, and more diverse representation of non-academic non-fiction domains,” she said.
The original Pile consists of 22 sub-datasets, including Books3 but also PubMed Central, Arxiv, Stack Exchange, Wikipedia, YouTube subtitles and, strangely, Enron emails. Biderman pointed out that the Pile remains the LLM training dataset most well-documented by its creator in the world. The objective in developing the Pile was to construct an extensive new data set, comprising billions of text passages, aimed at matching the scale of what OpenAI utilized for training GPT-3.
The Pile was a unique AI training dataset when it was released
“Back in 2020, the Pile was a very important thing, because there wasn’t anything quite like it,” said Biderman. At the time, she explained, there was one publicly available large text corpora, C4, which was used by Google to train a variety of language models.
“But C4 is not nearly as big as the Pile is and it’s also a lot less diverse,” she said. “It’s a really high-quality Common Crawl scrape.” (The Washington Post analyzed C4 in an April 2023 investigation which “set out to analyze one of these data sets to fully reveal the types of proprietary, personal, and often offensive websites that go into an AI’s training data.”)
Instead, EleutherAI sought to be more discerning and identify categories of information and topics that it wanted the model to know things about.
“That was not really something anyone had ever done before,” she explained. “75%-plus of the Pile was chosen from specific topics or domains, where we wanted the model to know things about it — let’s give it as much meaningful information as we can about the world, about things we care about.”
Skowron explained that EleutherAI’s “general position is that model training is fair use” for copyrighted data. But they pointed out that “there’s currently no large language model on the market that is not trained on copyrighted data,” and that one of the goals of the Pile v2 project is to attempt to address some of the issues related to copyright and data licensing.
They detailed the composition of the new Pile dataset to reflect that effort: It includes public domain data, both older works which have entered public domain in the US and text that was never within the scope of copyright in the first place, such as documents produced by the government or legal filings (such as Supreme Court opinions); text licensed under Creative Commons; code under open source licenses; text with licenses that explicitly permit redistribution and reuse — some open access scientific articles fall into this category; and a miscellaneous category for smaller datasets for which researchers have the explicit permission from the rights holders.
Criticism of AI training datasets became mainstream after ChatGPT
Concern over the impact of AI training datasets is not new. For example, back in 2018 AI researchers Joy Buolamwini and Timnit Gebru co-authored a paper that found large image datasets led to racial bias within AI systems. And legal battles began brewing over large image training datasets in mid-2022, not long after the the public began to realize that popular text-to-image generators like Midjourney and Stable Diffusion were trained on massive image datasets mostly scraped from the internet.
However, criticism of the datasets that train LLMs and image generators has amped up considerably since OpenAI’s ChatGPT was released in November 2022, particularly around concerns related to copyright. A rash of generative AI-focused lawsuits followed from artists, writers and publishers, leading up to the lawsuit that the New York Times filed against OpenAI and Microsoft last month, which many believe could end up before the Supreme Court.
But there have also been more serious, disturbing accusations recently — including the ease of creating deepfake revenge porn thanks to the large image corpora that trained text-to-image models, as well as the discovery of thousands child sexual abuse images in the LAION-5B image dataset — leading to its removal last month.
Debate around AI training data is highly-complex and nuanced
Biderman and Skowron say the debate around AI training data is far more highly-complex and nuanced than the media and AI critics make it sound — even when it comes to issues that are clearly disturbing and wrong, like the child sexual abuse images found in LAION-5B.
For instance, Biderman said that the methodology used by the people who flagged the LAION content are not legally accessible to the LAION organization, which she said makes safely removing the images difficult. And the resources to screen data sets for this kind of imagery in advance may not be available.
“There seems to be a very big disconnect between the way organizations try to fight this content and what would make their resources useful to people who wanted to screen data sets,” she said.
When it comes to other concerns, such as the impact on creative workers whose work was used to train AI models, “a lot of them are upset and hurt,” said Biderman. “I totally understand where they’re coming from that perspective.” But she pointed out that some creatives uploaded work to the internet under permissive licenses without knowing that years later AI training datasets could use the work under those licenses, including Common Crawl.
“I think a lot of people in the 2010s, if they had a magic eight ball, would have made different licensing decisions,” she said.
Still, EleutherAI also did not have a magic eight ball — and Biderman and Skowron agree when the Pile was created, AI training datasets were primarily used for research, where there are broad exemptions when it comes to license and copyright.
“AI technologies have very recently made a jump from something that would be primarily considered a research product and a scientific artifact to something whose primary purpose was for fabrication,” Biderman said. Google had put some of these models into commercial use in the back end in the past, she explained, but training on “very large, mostly web script data sets, this became a question very recently.”
To be fair, said Skowron, legal scholars like Ben Sobel had been thinking about issues of AI and the legal issue of “fair use” for years. But even many at OpenAI, “who you’d think would be in the know about the product pipeline,” did not realize the public, commercial impact of ChatGPT that was coming down the pike, they explained.
EleutherAI says open datasets are safer to use
While it may seem counterintuitive to some, Biderman and Skowron also maintain that AI models trained on open datasets like the Pile are safer to use, because visibility into the data is what helps the resulting AI models to be safely and ethically used in a variety of contexts.
“There needs to be much more visibility in order to achieve many policy objectives or ethical ideals that people want,” said Skowron, including thorough documentation of the training at the very minimum. “And for many research questions you need actual access to the data sets, including those that are very much of, of interest to copyright holders such as such as memorization.”
For now, Biderman, Skowron and their cohorts at EleutherAI continue their work on the updated version of the Pile.
“It’s been a work in progress for about a year and a half and it’s been a meaningful work in progress for about two months — I am optimistic that we will train and release models this year,” said Biderman. “I’m curious to see how big a difference this makes. If I had to guess…it will make a small but meaningful one.”
VentureBeat’s mission is to be a digital town square for technical decision-makers to gain knowledge about transformative enterprise technology and transact. Discover our Briefings.