This Paper Proposes Osprey: A Mask-Text Instruction Tuning Approach to Extend MLLMs (Multimodal Large Language Models) by Incorporating Fine-Grained Mask Regions into Language Instruction
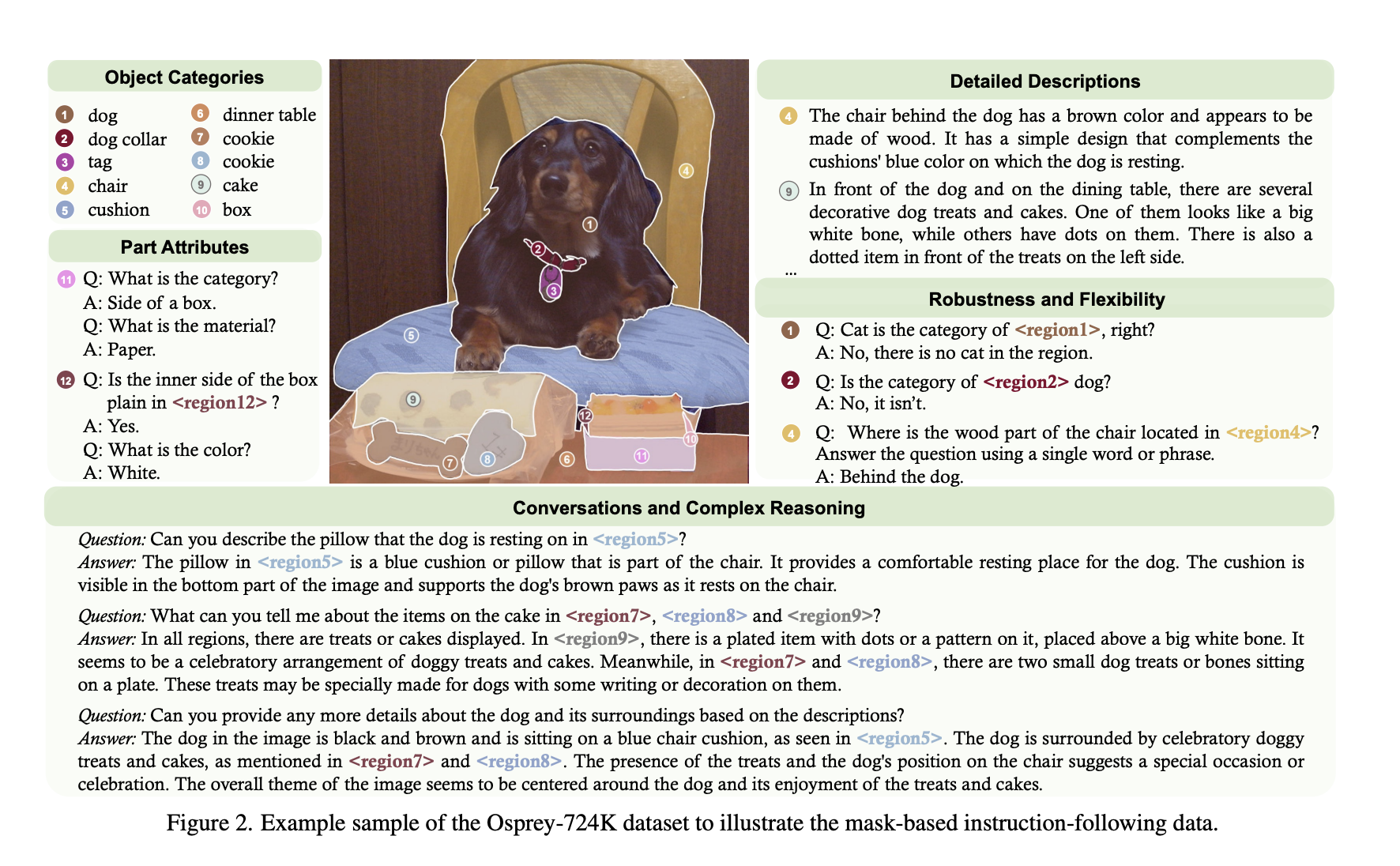
Multimodal Large Language Models (MLLMs) are pivotal in integrating visual and linguistic elements. These models, fundamental to developing sophisticated AI optical assistants, excel in interpreting and synthesizing information from text and imagery. Their evolution marks a significant stride in AI’s capabilities, bridging the gap between visual perception and language comprehension. The value of these models lies in their ability to process and understand multimodal data, a crucial aspect of AI applications in diverse fields like robotics, automated systems, and intelligent data analysis.
A central challenge in this field is the need for current MLLMs to achieve detailed vision-language alignment, particularly at the pixel level. Most existing models are proficient in interpreting images at a broader, more general level, using image-level or box-level understanding. While effective for overall image comprehension, this approach needs to improve in tasks that demand a more granular, detailed analysis of specific image regions. This gap in capability limits the models’ utility in applications requiring intricate and precise image understanding, such as medical imaging analysis, detailed object recognition, and advanced visual data interpretation.
The prevalent methodologies in MLLMs typically involve using image-text pairs for vision-language alignment. This approach is well-suited for general image understanding tasks but needs more finesse for region-specific analysis. As a result, while these models can effectively interpret the overall content of an image, they need help with more nuanced tasks such as detailed region classification, specific object captioning, or in-depth reasoning based on particular areas within an image. This limitation underscores the necessity for more advanced models capable of dissecting and understanding images at a much finer level.
Researchers from Zhejiang University, Ant Group, Microsoft, and The Hong Kong Polytechnic University have developed Osprey, an innovative approach designed to enhance MLLMs by incorporating pixel-level instruction tuning to address this challenge. This method aims to achieve a detailed, pixel-wise visual understanding. Osprey’s approach is groundbreaking, enabling a deeper, more nuanced understanding of images and allowing for precise analysis and interpretation of specific image regions at the pixel level.
At the core of Osprey is the convolutional CLIP backbone, used as its vision encoder, along with a mask-aware visual extractor. This combination is a key innovation, allowing Osprey to capture and interpret visual mask features from high-resolution inputs accurately. The mask-aware optical extractor can discern and analyze specific regions within an image with high precision, enabling the model to understand and describe these regions in detail. This feature makes Osprey particularly adept at tasks requiring fine-grained image analysis, such as detailed object description and high-resolution image interpretation.
Osprey has demonstrated exceptional performance and understanding of tasks across various regions. Its ability to excel in open-vocabulary recognition, referring object classification, and detailed region description is particularly noteworthy. The model showcases its capability to produce fine-grained semantic outputs based on class-agnostic masks. This capability indicates Osprey’s advanced proficiency in detailed image analysis, surpassing existing models’ ability to interpret and describe specific image regions with remarkable accuracy and depth.
In conclusion, the research can be summarized in the following points:
- The development of Osprey is a landmark achievement in the MLLM landscape, particularly addressing the challenge of pixel-level image understanding.
- The integration of mask-text instruction tuning with a convolutional CLIP backbone in Osprey represents a significant technological innovation, enhancing the model’s ability to process and interpret detailed visual information accurately.
- Osprey’s adeptness in handling tasks requiring intricate visual comprehension marks a crucial advancement in AI’s ability to engage with and interpret complex visual data, paving the way for new applications and advancements in the field.
Check out the Paper and Github. All credit for this research goes to the researchers of this project. Also, don’t forget to join our 35k+ ML SubReddit, 41k+ Facebook Community, Discord Channel, and Email Newsletter, where we share the latest AI research news, cool AI projects, and more.
If you like our work, you will love our newsletter..
Hello, My name is Adnan Hassan. I am a consulting intern at Marktechpost and soon to be a management trainee at American Express. I am currently pursuing a dual degree at the Indian Institute of Technology, Kharagpur. I am passionate about technology and want to create new products that make a difference.