Together AI Releases RedPajama v2: An Open Dataset with 30 Trillion Tokens for Training Large Language Models
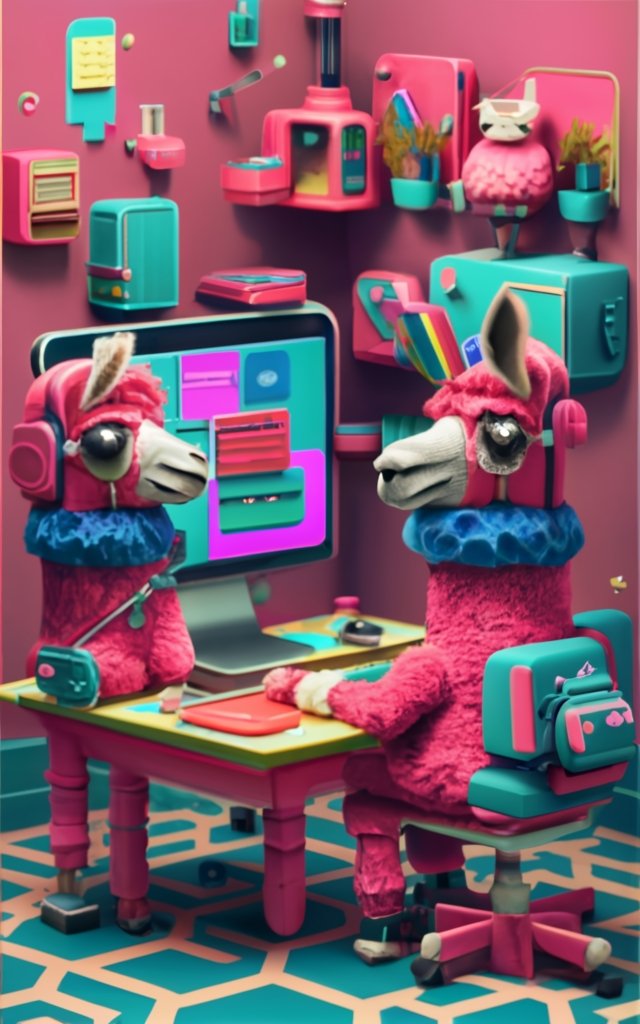
High-quality data are essential to the success of state-of-the-art open LLMs like Llama, Mistral, Falcon, MPT, and the RedPajama models. However, due to abnormalities emerging from the conversion of HTML to plain text, sources of generally low quality, and biases inherent in the diffusion of content on the web, this data is unrefined and not ideal for direct use in LLM training. Gathering the correct dataset and data mixture is a tedious task that requires a lot of time, resources, and money. Even though several community projects have been built up around this initiative, such as C4, RedPajama-1T, Refinedweb (Falcon), Dolma (AI2), and SlimPajama, many of these only cover a subset of the CommonCrawl crawls and offer a very narrow method of data filtering.
Researchers from Together.ai released RedPajama-1T in March this year, a 5TB dataset—more than 190,000 times and have been using them in imaginative ways. With 1 trillion high-quality English tokens, RedPajama-1T was just the beginning. The researchers have taken a step further by releasing RedPajama-V2, a vast, 30 trillion token online dataset, the largest publicly available dataset dedicated to learning-based machine-learning systems.
The team believes that RedPajama-Data-v2 will provide a repository of online data that can be used as a foundation for extracting high-quality datasets for LLM training and the foundation for in-depth study into LLM training data. They assert its coverage of CommonCrawl (84 processed dumps) is unparalleled. More crucially, they include 40+ quality annotations — the result of multiple ML classifiers on data quality, minhash results that may be used for fuzzy deduplication, or heuristics. An LLM developer may use these annotations to quickly and easily generate their custom pre-training dataset by slicing and filtering publicly available data.
CommonCrawl is the main emphasis of RedPajama-V2. RedPajama-V2 is constructed from the ground up using 84 CommonCrawl crawls and other publicly available web data. This dataset comprises raw data (plain text), 40+ high-quality annotations, and deduplication clusters.
Each CommonCrawl snapshot is first processed by the CCNet pipeline as the first step in assembling this dataset. Because of its minimal processing, this pipeline fits well with the overarching idea of keeping as much data in the raw form as feasible and letting model builders in the pipeline conduct their filtering and reweighting. Using CCNet’s language filter, we’ve only included English, French, Spanish, German, and Italian in this version. This stage of processing generates one hundred billion text pages.
For both the “head” and “middle” buckets, the researchers compute over 40 of the most popular quality annotations and the text documents processed by CCNet. The major goal of these annotations is to promote investigation into their optimal use and to enable model developers working downstream to filter or reweight the dataset according to their criteria. In addition, they hope to eventually add more high-quality signals with the community’s help.
Along with these minhash signatures, the team also do exact deduplication by applying a Bloom filter to the document’s sha1 hash digest. These are maintained as a separate quality annotation file to allow the original non-duplicated distribution to be restored to facilitate research in this approach.
RedPajama-v2 has 113B documents in English, German, French, Spanish, and Italian and is the result of processing 84 CommonCrawl crawls. The estimated 80B documents in the tail partition are retained, while the document and token counts in the head and middle partitions are determined before and after deduplication. The token count drops by 60%, but the number of documents drops by 71%, suggesting that the tail papers are typically shorter.
The dataset was reduced by around 40% after deduplicating the head+middle documents using a Bloom filter. The text documents provide the bulk of the dataset, including quality annotations and deduplication clusters. The layout is very similar to that specified by CCNet. To be more specific, each CommonCrawl snapshot’s pages are split into 5k shards, with the key indicating the shard, language, and perplexity bucket (partition).
The team hope to expand their current set of high-quality annotations soon to include things like contamination annotations compared to widely-used LLM benchmarks, topic modelling and categorization annotations for each document, and any additional annotations that spark interest in the community.
Check out the Github and Reference Blog. All Credit For This Research Goes To the Researchers on This Project. Also, don’t forget to join our 32k+ ML SubReddit, 40k+ Facebook Community, Discord Channel, and Email Newsletter, where we share the latest AI research news, cool AI projects, and more.
If you like our work, you will love our newsletter..
We are also on Telegram and WhatsApp.
Dhanshree Shenwai is a Computer Science Engineer and has a good experience in FinTech companies covering Financial, Cards & Payments and Banking domain with keen interest in applications of AI. She is enthusiastic about exploring new technologies and advancements in today’s evolving world making everyone’s life easy.