Unlocking the Brain’s Language Response: How GPT Models Predict and Influence Neural Activity
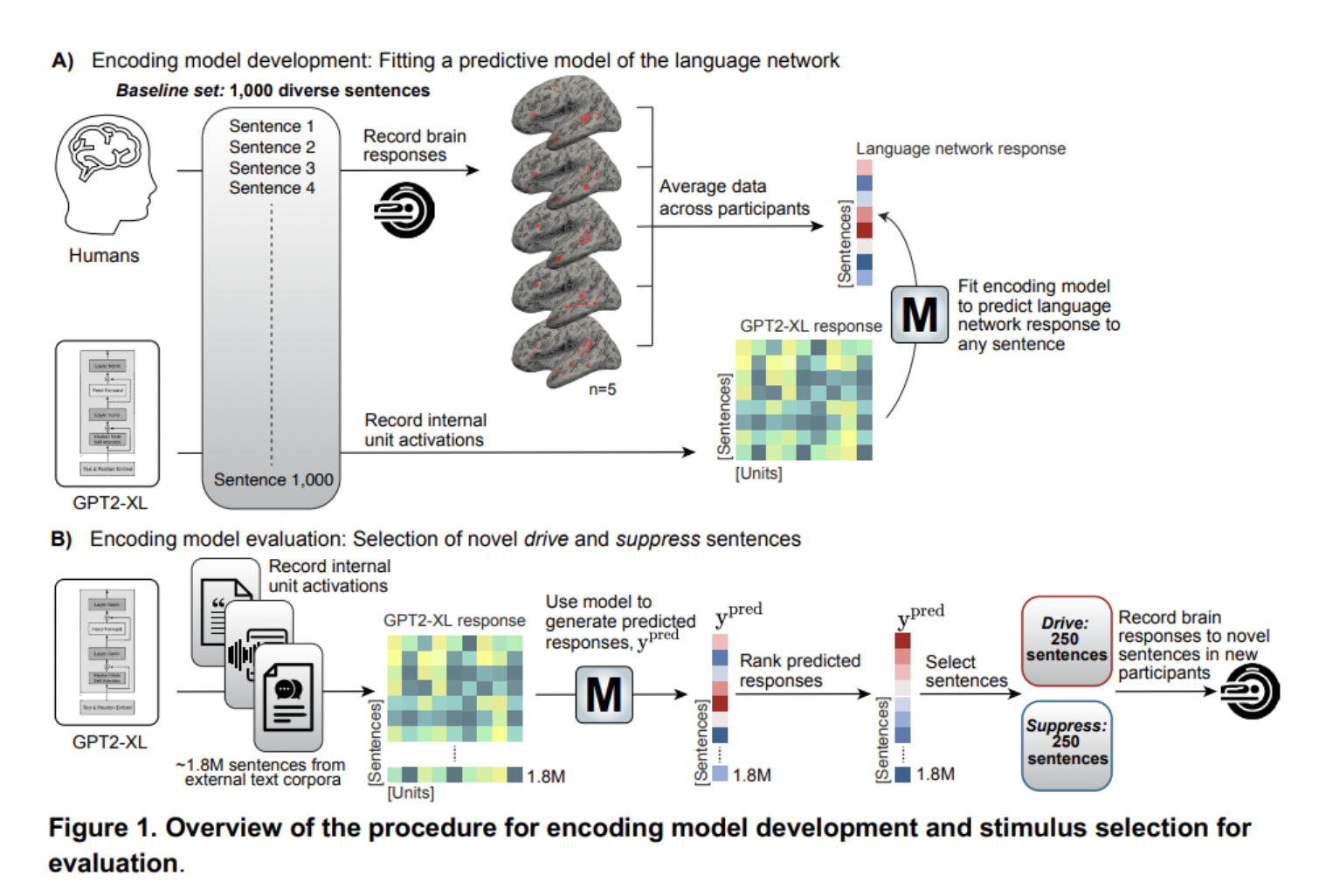
Recent advancements in machine learning and artificial intelligence (ML) techniques are used in all fields. These advanced AI systems have been made possible due to advances in computing power, access to vast amounts of data, and improvements in machine learning techniques. LLMs, which require huge amounts of data, generate human-like language for many applications.
A new study by researchers from MIT and Harvard University have developed new insights to predict how the human brain responds to language. The researchers emphasized that this can be the first AI model to effectively drive and suppress responses in the human language network. Language processing involves language networks, specifically brain areas primarily in the left hemisphere. They include parts of the frontal and temporal lobes of the brain. There has been research to understand how this network functions, but much is still to be known about the underlying mechanisms involved in language comprehension.
Through this study, the researchers tried to evaluate LLMs’ effectiveness in predicting brain responses to various linguistic inputs. Also, they aim to understand better the characteristics of stimuli that drive or suppress responses within the language network area of humans. The researchers formulated an encoding model based on a GPT-style LLM to predict the human brain’s reactions to arbitrary sentences presented to participants. They built this encoding model using last-token sentence embeddings from GPT2-XL. It was trained on a dataset of 1,000 diverse, corpus-extracted sentences from five participants. Finally, they tested the model on held-out sentences to assess its predictive capabilities. They concluded that the model achieved a correlation coefficient of r=0.38.
To further evaluate the model’s robustness, the researchers performed several other tests using alternative methods for obtaining sentence embeddings and incorporating embeddings from another LLM architecture. They found that the model maintained high predictive performance in these tests. Also, they found that the encoding model was accurate for predictive performance when applied to anatomically defined language regions.
The researchers emphasized that this study and its findings hold substantial implications for fundamental neuroscience research and real-world applications. They noted that manipulating neural responses in the language network can open new fields for studying language processing and potentially treating disorders affecting language function. Also, implementing LLMs as models of human language processing can improve natural language processing technologies, such as virtual assistants and chatbots.
In conclusion, this study is a significant step in understanding the connection and working similarity between AI and the human brain. Researchers use LLMs to unravel the mysteries surrounding language processing and develop innovative strategies for influencing neural activity. Researchers expect to see more exciting discoveries in this domain as AI and ML evolve.
Check out the Paper. All credit for this research goes to the researchers of this project. Also, don’t forget to follow us on Twitter and Google News. Join our 36k+ ML SubReddit, 41k+ Facebook Community, Discord Channel, and LinkedIn Group.
If you like our work, you will love our newsletter..
Don’t Forget to join our Telegram Channel
Rachit Ranjan is a consulting intern at MarktechPost . He is currently pursuing his B.Tech from Indian Institute of Technology(IIT) Patna . He is actively shaping his career in the field of Artificial Intelligence and Data Science and is passionate and dedicated for exploring these fields.