Vector database company Qdrant wants RAG to be more cost-effective
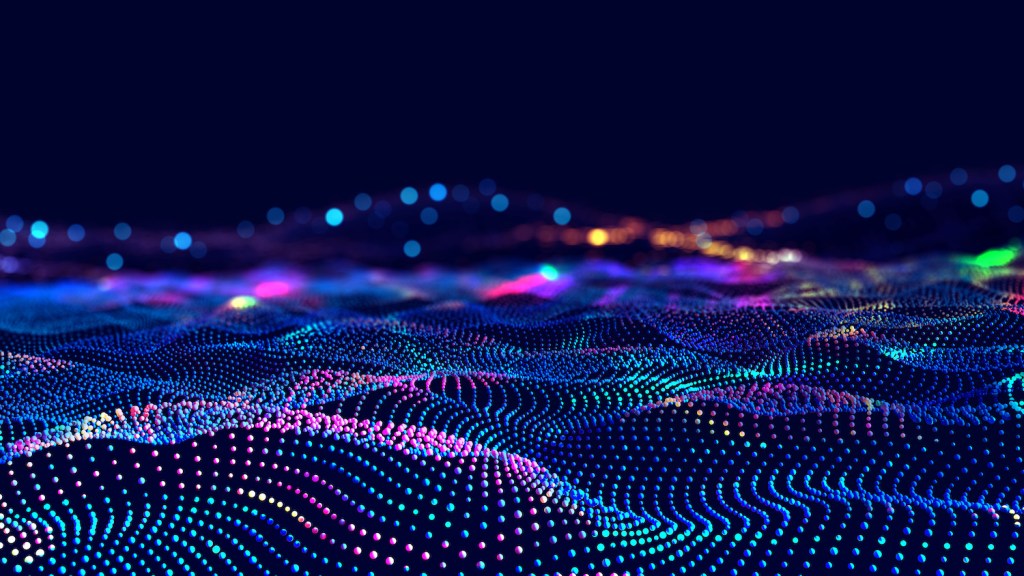
Don’t miss OpenAI, Chevron, Nvidia, Kaiser Permanente, and Capital One leaders only at VentureBeat Transform 2024. Gain essential insights about GenAI and expand your network at this exclusive three day event. Learn More
More companies are looking to include retrieval augmented generation (RAG) systems in their technology stack, and new methods to improve it are now coming to light.
Vector database company Qdrant believes its new search algorithm, BM42, will make RAG more efficient and cost-effective.
Qdrant, founded in 2021, developed BM42 to provide vectors to companies working on new search methods. The company wants to offer more hybrid search—which combines semantic and keyword search—to customers.
Andrey Vasnetsov, co-founder and chief technology officer of Qdrant, said in an interview with VentureBeat that BM42 is an update to the algorithm BM25, which “traditional” search platforms use to rank the relevance of documents in search queries. RAG often uses vector databases or databases that store data as mathematical metrics that make it easy to match data.
Countdown to VB Transform 2024
Join enterprise leaders in San Francisco from July 9 to 11 for our flagship AI event. Connect with peers, explore the opportunities and challenges of Generative AI, and learn how to integrate AI applications into your industry. Register Now
“When we apply traditional keyword matching algorithms, the most commonly used one is BM25, which assumes documents have enough size to calculate statistics,” Vasnetsov said. “But we’re working with chunks of information now with RAG, so it doesn’t make sense to use BM25 anymore.”
Vasnetsov added that BM42 uses a language model, but instead of creating embeddings or representations of information, the model extracts the information from the documents. This information becomes tokens, which the algorithm then scores or weights in order to rank its relevance to the search question. This lets Qdrant pinpoint the exact information needed to answer a query.
Hybrid search has many options
However, BM42 is not the first method to look to overtake BM25 to make it easier to do hybrid research and RAG. One such option is Splade, which stands for Sparse Lexical and Expansion model.
It works with a pre-trained language model that can identify relationships between words and include related terms that may not be the same between the search query text and the documents it references.
While other vector database companies use Splade, Vasnetsov said BM42 is a more cost-efficient solution. “Splade can be very expensive because these models tend to be really huge and require a lot of computation. So it’s still expensive and slow,” he said.
RAG is quickly becoming one of the hottest topics in enterprise AI, as companies want a way to use generative AI models and map these to their own data. RAG could bring more accurate and real-time information from company data to employees and other users.
Companies like Microsoft and Amazon now offer infrastructure for cloud computing clients to build RAG applications. In June, OpenAI acquired Rockset to beef up its RAG capabilities.
But while RAG lets users ground the information AI models read to company data, it is still a language model that can be prone to hallucinations.