Microsoft Researchers Introduce Kosmos-2.5: A Multimodal Literate Model for Machine Reading of Text-Intensive Images
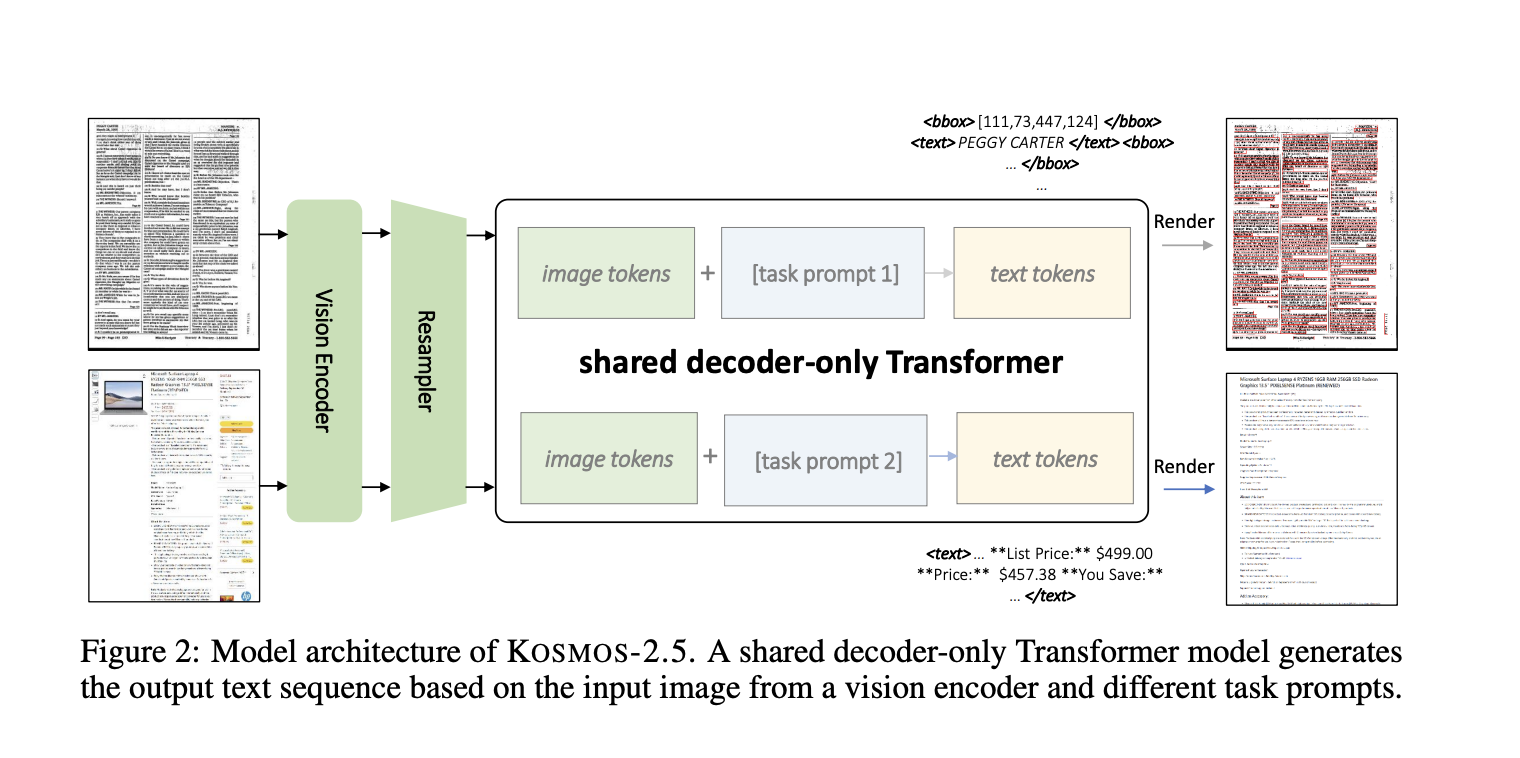
In recent years, large language models (LLMs) have gained prominence in artificial intelligence, but they have mainly focused on text and struggled with understanding visual content. Multimodal large language models (MLLMs) have emerged to bridge this gap. MLLMs combine visual and textual information in a single Transformer-based model, allowing them to learn and generate content from both modalities, marking a significant advancement in AI capabilities.
KOSMOS-2.5 is a multimodal model designed to handle two closely related transcription tasks within a unified framework. The first task involves generating text blocks with spatial awareness and assigning spatial coordinates to text lines within text-rich images. The second task focuses on producing structured text output in markdown format, capturing various styles and structures.
Both tasks are managed under a single system, utilizing a shared Transformer architecture, task-specific prompts, and adaptable text representations. The model’s architecture combines a vision encoder based on ViT (Vision Transformer) with a language decoder based on the Transformer architecture, connected through a resampler module.
To train this model, it undergoes pretraining on a substantial dataset of text-heavy images, which include text lines with bounding boxes and plain markdown text. This dual-task training approach enhances KOSMOS-2.5’s overall multimodal literacy capabilities.
The above image shows the Model architecture of KOSMOS-2.5. The performance of KOSMOS-2.5 is evaluated across two main tasks: end-to-end document-level text recognition and the generation of text from images in markdown format. Experimental results have showcased its strong performance in understanding text-intensive image tasks. Additionally, KOSMOS-2.5 exhibits promising capabilities in scenarios involving few-shot and zero-shot learning, making it a versatile tool for real-world applications that deal with text-rich images.
Despite these promising results, the current model faces some limitations, offering valuable future research directions. For instance, KOSMOS-2.5 currently does not support fine-grained control of document elements’ positions using natural language instructions, despite being pre-trained on inputs and outputs involving the spatial coordinates of text. In the broader research landscape, a significant direction lies in furthering the development of model scaling capabilities.
Check out the Paper and Project. All Credit For This Research Goes To the Researchers on This Project. Also, don’t forget to join our 30k+ ML SubReddit, 40k+ Facebook Community, Discord Channel, and Email Newsletter, where we share the latest AI research news, cool AI projects, and more.
If you like our work, you will love our newsletter..
Janhavi Lande, is an Engineering Physics graduate from IIT Guwahati, class of 2023. She is an upcoming data scientist and has been working in the world of ml/ai research for the past two years. She is most fascinated by this ever changing world and its constant demand of humans to keep up with it. In her pastime she enjoys traveling, reading and writing poems.