Stephen Wolfram Talks Intelligence And Large Language Models
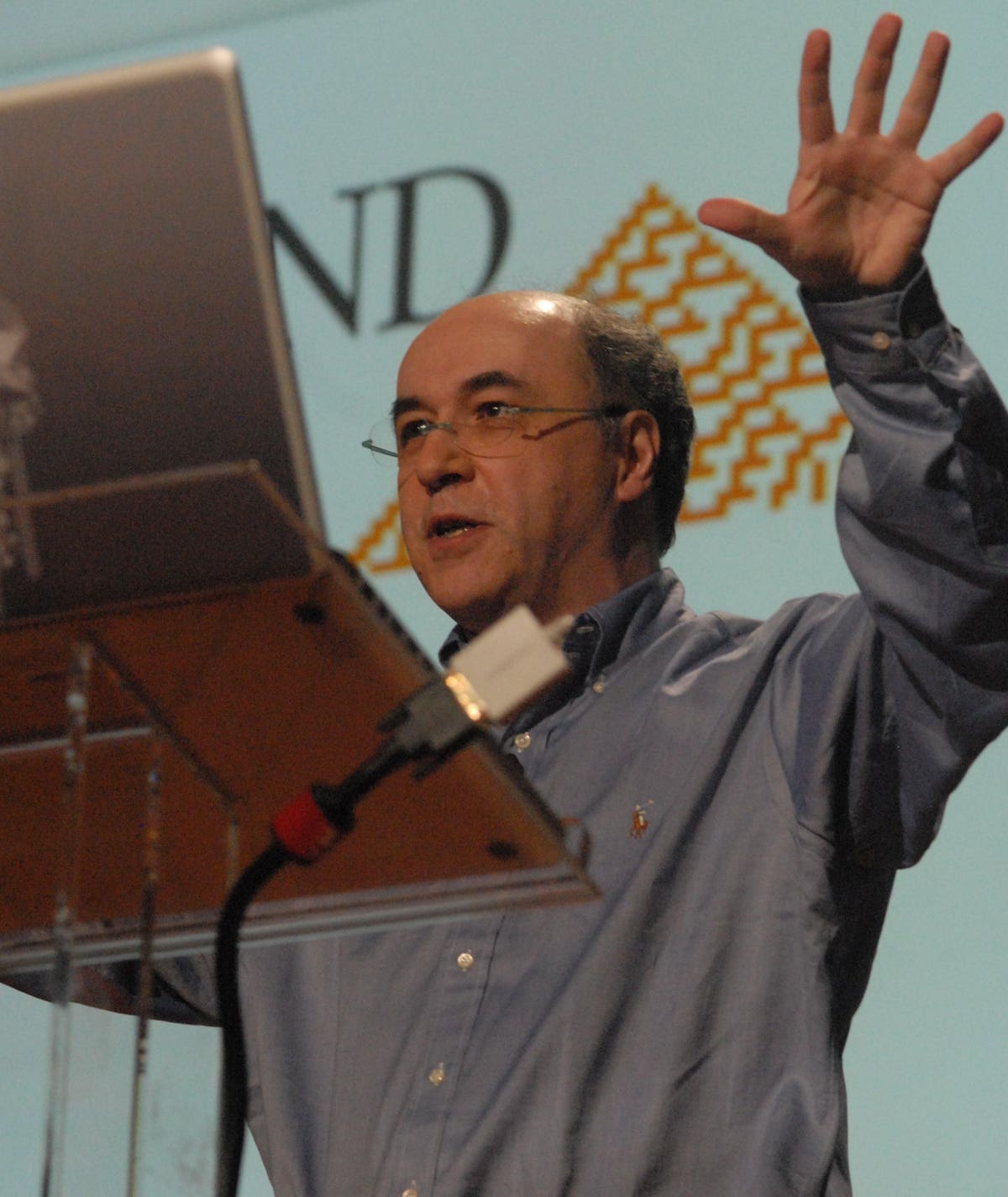
They’re two great tastes that taste great together. Or rather, they’re two technologies that, put together in collaborative ways, are becoming much more powerful!
Wolfram
John Werner
Marvin Minsky famously said that the brain is not one computer, but several hundred computers working in tandem. If that’s true, ChatGPT’s cognitive power just got a boost with the creation of a Wolfram Alpha plug-in that allows for the two systems to send and receive natural language input, so that ChatGPT systems can utilize a different system of symbolic representation that had already been pioneered before the days when we could just ask a computer to write an essay.
We heard early this year that teams were working on this merge, and it’s been interesting to the AI community. Now it’s come to fruition.
Stephen Wolfram talks about the universal linguistic user interface, Wolfram Alpha’s creation in 2009, and what’s in store for the future.
In starting out talking about the progress that we’ve made with LLMs and related tech, Wolfram makes the analogy to electricity and all of its modern use cases. Right now, he suggests, we’re figuring out all of those applications of AI and large language models. So what are we seeing? Wolfram mentions a search for the “concrete meaning for the concept of understanding” and explains that through “rich experiences,” we are all getting more of a front-row seat to how this sort of computational power works.
“There are (cases where) you can compute, and you can go on computing, and you can figure out things that are very different from what we humans can figure out,” he says. “And that’s what I’ve spent a lot of time building – the ability to do deep computation, and the way to … connect (that) computation to things that we humans can talk about. And so the thing that I consider pretty interesting right now, is that ChatGPT provides this linguistic user interface (and) we can now connect to deep computation.”
One thing that Wolfram talks about a good bit is the concept of democratized access. We’ve seen a lot of self-service applications of AI, and he suggests new models are going to bring this much further. In talking about the idea of what computation does that sort of mundane, and what it does that’s fairly amazing, he introduces the “regularity of language” as something that humans could explore for themselves. On the other hand, he mentions the principle of “computational irreducibility” that shows the true power of computation.
“We can do a little bit of math in our heads,” he says. “I don’t know that many people who can run programs in their heads. That’s a really hard thing to do. But yet computers manage to run programs all the time. And it’s the question of: how do we get access to that sort of power of computation?”
Most fields of human endeavor, he says, take advantage of certain information structures. The idea is that new computing systems can create those structures in a way that we couldn’t do in our own minds, but, on the other hand, in a way that we can follow, at least in theory.
He describes building on current technologies as generating a sort of new form of mathematical notation that humans can better understand, and the power of interfaces like ChatGPT to make results digestible to human users.
Later, Wolfram also makes another important contrast: to systems in nature that can be sentient in their own ways, without thinking in the same ways that humans do.
If you see this part of the video where he talks about the weather “having a mind of its own,” Wolfram is coming back to this subtlety in understanding how these new technologies have their own kinds of understandings that are not ours!
We can do sophisticated things and create progress, he says, in an explainable way, due to the power of these technologies, but we can do more if we understand how they think. While he points out that you need to define what you want to do in designing a workflow, he also notes that this kind of work is going to become more valuable in the future.
He also contrasts the traditional practice of programming to the newer practice of using a computational language in a different, less deterministic way.
“A programming language is something which kind of tells the computer in its terms, what to do,” he says, “you know, set this variable to this, make this loop … things like that. The idea of a computational language (involves describing) the world computationally in a form that we humans can understand, talking about cities, and chemicals, and images and whatever else, not (just) talking about the things that are intrinsic to a computer, (but) having a specification, this computational specification of what you want.”
Instead of learning to program, he says, people should be learning to think about things in a computational way. With that in mind, he goes over the imperative of figuring out what AI will automate, and what it won’t.
Here’s where decision support comes in – Wolfram suggests that, unlike what he characterizes as a hidebound “industrial” style education system that we have, in the future world of learning, people will not be learning to find the right answers, but instead, to ask the right questions.
He talks about using assembly language in prior decades, and how that’s obsolete now. Should you learn to program? Wolfram contends that it will be more useful to learn to make use of high-level interfaces and to generalize away from a particular code syntax. Though noting that “prompt engineering is a very weird business,” he predicts that in the years to come, it’s going to be a big business, too.
Wolfram also makes an interesting point on the nature of human language and human psychology – you need to stay with him for a while to hear it, but it sheds some interesting light on how the history books might describe our current forays into AI.
He first suggests that maybe humans should have been able to understand the semantic nature of language better before ChatGPT came on the scene. Was it a missed opportunity? Then he suggests that human psychology might end up being a lot like human language. When computers start to explore it, they might teach us new things that are going to be sort of embarrassing in terms of their newness.
That in itself is a pretty intimidating picture of how humans stack up to machines, but Wolfram’s recommendation that humans learn broadly at least sets us up to take advantage of AI as decision support, in other words, to bring our human capability for decision making to the table. That’s a principle that we already have, to some extent, but it’s going to help us navigate the new world where robots and computers are, well, pretty smart…
Near the end of the video, Wolfram talks about the development story for putting Wolfram Alpha and ChatGPT together, and while you can see this headlined in news pieces over the past few months, here you get insight from someone with skin in the game.
At the beginning, he says, engineers and other stakeholders didn’t know if the plan would work, but in the end, they realized that there was a sort of natural API in Wolfram Alpha’s ability to use natural language input.
“I felt pretty good about it,” he said of the process in general.
Wolfram said it only took a few weeks for a number of good teams to put these things together and blend two possible plug-ins into one.
“That’s not a trivial thing,” he said.
Last, but not least, Wolfram suggested new things are coming.
Specifically, he talked about new research to take the ‘notebook paradigm’ inherent in WA and generalize it to the chat world.
What that sounds like is that we’re going to be applying language models in greater numbers of conversational ways.