This AI Paper Unveils OpenBA: An Open-Sourced 15B Parameter Bilingual Model Outperforming Predecessors and Aiding Chinese-centric NLP Advancements
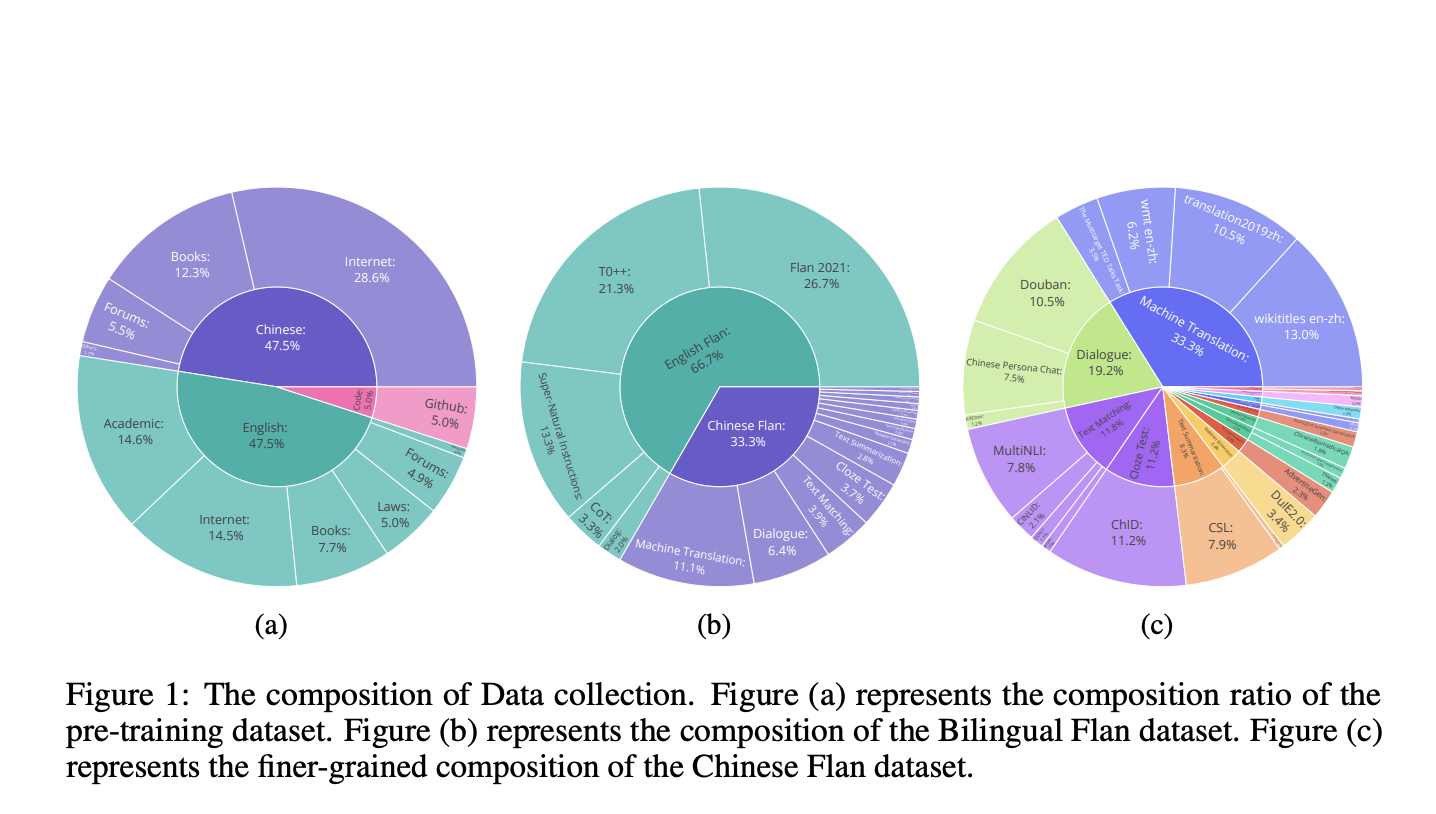
The scaling rule of language models has produced success like never before. These huge language models have gotten novel emerging capabilities in addition to demonstrating tremendous superiority over earlier paradigms for many disciplines when trained on immense amounts of textual data. Although highly strong and evolving quickly, these models at scale still need to be ideal or sufficient for most real-world applications. The open-source community has worked hard to provide robust and openly accessible LLMs that cover a variety of data sources, architectures, language modeling objectives, training pipelines, model scales, and languages of expertise, such as BLOOM, LLaMA, FlanT5, and AlexaTM.
Chinese-LLaMA, MOSS, Huatuo, Luotuo, and Phoenix are some of the numerous big language models made available by the open-source community, either by pre-training from scratch or by further fine-tuning existing multilingual models. Strong general language models and various decoder-only variants are made available to researchers and developers by these publicly accessible LLMs. Still, the Encoder-Decoder framework remains under-explored, which is universally effective for multiple tasks, including language comprehension, common sense reasoning, question-and-answering, information retrieval, and multi-turn chit-chat conversations.
Researchers from Soochow University contribute an open-sourced 15B bilingual asymmetric seq2seq model (OpenBA) that has been pre-trained from scratch to fill this gap, providing not only the model checkpoints but also the data collection and processing information to create the pre-training data and bilingual Flan collection from freely available data sources (such as Common Crawl, the Pile corpus, and C-Book), the motivations and empirical observations for the model architecture design, and the key information of other enhanced models. They specifically gathered pre-training data balanced between English and Chinese tokens to assist the Chinese language modeling. They include additional English data from the Flan collection in their Bilingual-Flan corpus since it is challenging to create a Chinese collection similar to Flan that covers a wide range of jobs and environments using just available resources.
They use a different asymmetric model structure, namely a shallow-encoder deep decoder, to improve the generation capability. This differs from the vanilla Flan-T5 of a balanced encoder-decoder structure and the asymmetric deep-encoder shallow-decoder in AlexaTM. The three stages of their training procedure are the UL2 pre-training, length-adaptation, and Flan training. They also apply enhancement tactics to model architecture and training to enhance model capacity, stability, and effectiveness. The efficacy of their model has been shown in tests using a variety of benchmarks (MMLU, CMMLU, C-Eval, SuperGLUE, BELEBELE, and BBH) and tasks (such as understanding, reasoning, and generating). These tests also included zero-shot, few-shot, held-in, and held-out settings.
Their model can outperform numerous typical models, such as LLaMA-70B on BELEBELE, BLOOM-176B on MMLU, ChatGLM-6B on CMMLU, and C-Eval, despite having just been trained on 380B tokens. Compared to the LLaMA-7B model, which uses 14 tCO2eq throughout the training phase, OpenBA-15B uses just about 6.5 tCO2eq overall. All implementation-related information, including data collection and processing, codes, model checkpoints, and assessments, is publicly available. They encourage any feedback and recommendations as they continue to work on ways to enhance and implement the OpenBA paradigm, and they look forward to continuing their collaboration with the open-source community.
Check out the Paper and Github. All Credit For This Research Goes To the Researchers on This Project. Also, don’t forget to join our 30k+ ML SubReddit, 40k+ Facebook Community, Discord Channel, and Email Newsletter, where we share the latest AI research news, cool AI projects, and more.
If you like our work, you will love our newsletter..
Aneesh Tickoo is a consulting intern at MarktechPost. He is currently pursuing his undergraduate degree in Data Science and Artificial Intelligence from the Indian Institute of Technology(IIT), Bhilai. He spends most of his time working on projects aimed at harnessing the power of machine learning. His research interest is image processing and is passionate about building solutions around it. He loves to connect with people and collaborate on interesting projects.